Big data for small earthquakes: Data mining, deep learning and explainable AI
No webcast
This seminar is not available to view online.
Karianne Bergen
Brown University
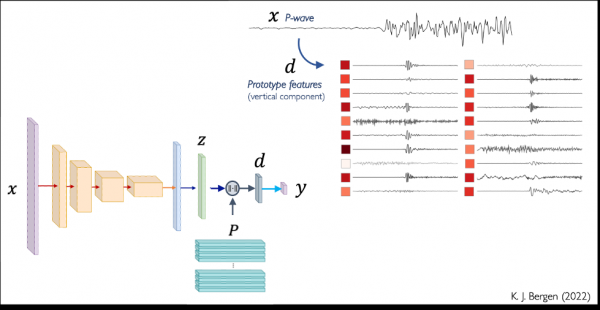
- Date & Time
- Location
- Online-only seminar via Microsoft Teams
- Host
- Shanna Chu
- Summary
Geophysicists are increasingly adopting algorithmic advances from machine learning (ML) and data mining techniques to advance the state-of-the-art in seismic signal analysis for earthquake monitoring.
One example is FAST (Yoon et al, 2015), a method for detecting similar waveforms (candidate earthquakes) in large-scale continuous seismic data when template waveforms are unavailable. Another example are deep neural networks, which have emerged as particularly powerful tools for classifying waveforms and identifying seismic phases. These models can learn complex patterns and decision functions with high predictive accuracy. But the complexity and large number of parameters also make these “black box” models difficult to inspect or interpret. The emerging field of explainable artificial intelligence (XAI) is developing tools and techniques to enable ML solutions that can be understood by human analysts. This work presents an interpretable one-dimensional convolutional neural network (CNN) model for earthquake phase detection. In this approach, a simple CNN-based detector (Ross et al.,2018) is modified to learn representative waveforms for classification. These prototype waveforms are used directly by the model to generate the predictions and can be used to explain the model outputs. The new prediction model achieves high prediction accuracy and has a similar architecture to the original CNN model, with the added benefit of greater interpretability. I will conclude by highlighting how research in the emerging discipline of scientific machine learning will play a critical role in driving discovery in the Earth and physical sciences.