Deep Learning for Earthquake Monitoring
No webcast
This seminar is not available to view online.
Weiqiang Zhu
UC Berkeley
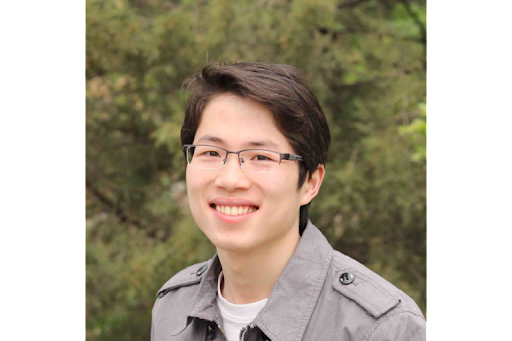
- Date & Time
- Location
- Online via Microsoft Teams and Moffett Bldg 19 Yosemite Room
- Host
- Shanna Chu
- Summary
Seismic networks are continuously improving in density and coverage, resulting in exponentially growing datasets of seismic waveforms. This large seismic archive poses a challenge for data analysis but also presents an opportunity to study many hidden earthquake signals such as small earthquakes that are missing from routine catalogs. These small earthquakes occur on the same faults as large earthquakes and may share similar physical mechanisms. Thus, we can extract important information from improved earthquake catalogs to study fault zone structures, earthquake physics, and related subsurface processes like fluid migration. Recent developments of deep learning have significantly improved earthquake monitoring, enabling rapid and accurate detection of many more small earthquakes with better constrained source parameters. In this talk, I will present our work on developing a deep-learning-based earthquake monitoring workflow using both seismic networks and distributed acoustic sensing (DAS). We applied the new deep-learning-based workflow to studying complex earthquake sequences including tectonic, induced, and volcanic earthquakes.
Closed captions are typically available a few days after the seminar. To turn them on, press the ‘CC’ button on the video player. For older seminars that don’t have closed captions, please email us, and we will do our best to accommodate your request.