Predicting the instantaneous and future laboratory fault slips through deep learning
Kun Wang
Geophysics Group & Center for Nonlinear Studies, LANL
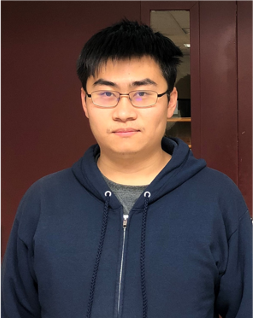
- Date & Time
- Location
- Online-only seminar via Microsoft Teams
- Host
- Tim Clements
- Summary
The previous 5 years demonstrate that machine learning is a powerful tool for studying laboratory faults using acoustic emissions (AE) that broadcast from the sliding interface. The AEs can inform us of the instantaneous fault frictional state in shear experiments and also contain information regarding the time remaining to the upcoming laboratory earthquake
(frictional failure). We present a deep learning approach using transfer learning for predicting the instantaneous status of the laboratory fault, with a unique emphasis on learning from extremely limited data. Although large training data sets are available from numerical simulations and
laboratory experiments, in Earth earthquake interevent times range from 10’s-100’s of years and continuous geophysical data typically exist for only a portion of an earthquake cycle. If the goal is predicting slip on seismogenic faults in Earth, then sparse data presents a serious challenge for applying machine learning. Hence, we developed a prototype transfer learning approach using fault-slip numerical simulations and laboratory data to address the sparse data situation. A convolutional encoder-decoder (CED) model learns a mapping between AE histories and fault friction from rich numerical simulations data. The model’s latent space is further trained using data from only a few laboratory-event earthquake-cycles, and even a small portion of pre- or post- failure data as an analog to a fault in Earth where data is sparse. Model predictions markedly improve by further training the model latent space and elucidate the potential of using machine learning models trained on numerical simulations and fine-tuned with small geophysical data sets for potential applications to faults in Earth. Furthermore, we address the open question
on whether the AE from laboratory experiments contains near-future frictional information. The approach applies a CED model and a self-attention transformer model that uses AE histories to predict the upcoming frictional behavior. Notably, information for predicting near future frictional failure and recovery are found to be contained in the AE signal, but when looking farther into the future the predictions are progressively worse. This first effort predicting future fault frictional behavior with machine learning will guide efforts for applications in Earth.
Closed captions are typically available a few days after the seminar. To turn them on, press the ‘CC’ button on the video player. For older seminars that don’t have closed captions, please email us, and we will do our best to accommodate your request.